Developments in Computational Finance and Stochastic Numerics - Honoring John Schoenmakers on the occasion of his retirement
Weierstrass Institute Berlin, July 01, 2024
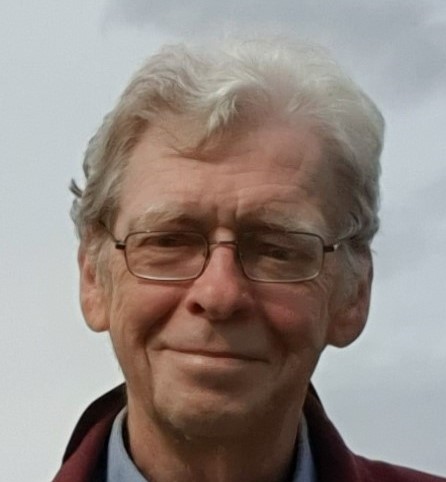
Overview
Important topics by the last turn of the century were advanced methods for interest rate modeling and pricing of complexly structured interest rate products. From a mathematical point of view such methods translate to numerically solving multidimensional Stochastic Differential Equations and solving problems of optimal stopping and control. In this field John and his coauthors contributed with various new methods ranging from stochastic policy iteration for single and multiple stopping to new dual martingale representations and regression methods for multiple stopping and control. For example, they were the first who circumvented the (until then) thorny need for nested Monte Carlo simulations for computing (dual) upper bounds for American options, by incorporating a regression approach inspired by Malliavin Calculus. Other strands of research where John Schoenmakers actively contributed were affine processes, multilevel Monte Carlo, generalized risk measures, McKean-Vlasov models, rough paths, and more.
John Schoenmakers holds a PhD in theoretical probability from Delft University (the Netherlands) in the field of stochastic processes. After his PhD he turned to more applied probabilistic topics such as environmental modeling and later on mainly to computational finance. Since 1998 he is the deputy head of the research group Stochastic Algorithms and Nonparametric Statistics at the Weierstrass Institute Berlin, in charge of the development and analysis of stochastic algorithms with particular focus on financial mathematical applications relevant for the financial industry.
For more information, please visit John's website.
Invited speakers
|
Organizers
|
Program
09:00 - 09:30 | Registration | 09:30 - 09:45 | Michael Hintermüller (WIAS Berlin) Welcome | ||
09:45 - 10:25 | Peter Kloeden (Goethe University Frankfurt/Main) Euler-like numerical schemes for Caputo fractional differential equations: deterministic and stochastic (abstract)Explicit and implicit Euler schemes are formulated for Caputo fractional differential equations (for short Caputo FDEs) of order α ∈ (0,1) whose vector fields satisfy a standard Lipschitz continuity condition in the state variable and a Hölder continuity condition in the time variable. The convergence rates of these schemes are given investigated. Stochastic counterparts are also considered. The corresponding equations do not satisfy the Itô formula. |
||||
10:25 - 10:55 | Coffee break | ||||
10:55 - 11:35 | Arnold W. Heemink (Delft University of Technology) Reverse time diffusion revisited: tracing water masses in the ocean (abstract)Particle models are often used to simulate pollution transport. More than 15 years ago, John Schoenmakers, Grigori Milstein, Arnold Heemink and Daria Spyvakovskaya, a PhD student at Delft University of Technology, used the concept of reverse time diffusion to obtain an efficient forward-reverse estimator for solving specific pollution problems (Advances in Water Research, 2005). More than 10 years later, the methodology, surprisingly, turned out to be very useful for ocean dynamics problems too: Tracing the ventilation pathways of oceans (Journal of Physical Oceanography, 2017). In the presentation, the important contributions of John Schoenmakers to the methodology will be addressed, and a number of practical applications in pollution transport and ocean dynamics will be used to illustrate the methodology. |
||||
11:35 - 12:15 | Christian Bender (Saarland University Saarbruecken) Regression Anytime (abstract)Fifteen years ago, John, Denis Belomestny, and I published a non-nested Monte Carlo algorithm for computing dual upper bounds for Bermudan option prices. The key idea of this method is to come up with a regression estimator for the Doob martingale part of the approximative Snell envelope, which is based on the martingale representation theorem and, thus, preserves the martingale property. In this talk, we discuss an algorithm which combines the advantages of regression-now algorithms (generic applicability) and regression-later algorithms (vanishing variance) for approximating the integrand in the martingale representation theorem via simulation-based least-squares regression. More generally, the algorithm can be applied to a class of regression problems with Malliavin weights. Our error analysis shows that the convergence in the number of Monte Carlo samples can take place at an arbitrarily fast polynomial rate, if the problem is sufficiently smooth. The talk is based on joint work with Nikolaus Schweizer (Tilburg). |
||||
12:15 - 13:30 | Lunch break | ||||
13:30 - 14:10 | Roger Laeven (University of Amsterdam) Saddlepoint Approximations for Hawkes Jump-Diffusion Processes with an Application to Risk Management (abstract)We propose a statistical model based on Hawkes processes in which large financial losses can arise in close succession serially as well as cross-sectionally. We derive in closed form saddlepoint approximations to the tails of profit and loss distributions, both marginal and joint, and use them to construct explicit risk measure formulae that account for the fact that a given financial institution's losses make it more likely that that institution will experience further losses, and that other financial institutions will experience losses as well. These closed-form risk measures can be used for comparative statics, parameter calibration, and setting capital requirements and potential systemic risk charges. (Based on joint work with Yacine Aït-Sahalia.) |
||||
14:10 - 14:50 | Dennis Belomestny (Duisburg-Essen University) Preference-based reinforcement learning (abstract)Reinforcement learning (RL) algorithms are designed to maximize the cumulative reward according to a carefully chosen reward function. However, creating such a reward function often requires task-specific prior knowledge, which may not be available in a quantifiable form. To address these challenges, preference-based reinforcement learning algorithms have been developed. These algorithms can learn directly from an experts preferences rather than relying on a manually designed numerical reward function. In this presentation, I will provide an overview of preference-based reinforcement learning and demonstrate its core principles using examples. Specifically, I will discuss the types of human feedback that can be assumed and how preferences can be incorporated into the optimization problem through penalization. |
||||
14:50 - 15:20 | Coffee break | ||||
15:20 - 16:00 | Martin Redmann (Martin Luther University of Halle) Solving high-dimensional problems in finance using model reduction (abstract)We consider high-dimensional asset price models that are reduced in their dimension in order to lower the computational complexity or the effect of the curse of dimensionality in the context of option pricing. We apply model order reduction to obtain a reduced system and use concepts known for deterministic control systems. We sketch different schemes leading to a good pathwise approximation of the underlying. The error of the approximation is analyzed and the performance of the algorithm is shown conducting several numerical experiments. |
||||
16:00 - 16:40 | Volker Krätschmer (Duisburg-Essen University) Nonasymptotic upper estimate for errors of the sample average approximation method to solve risk averse stochastic programs (abstract)We study statistical properties of the optimal value of the Sample Average Approximation. The focus is on the tail function of the absolute error induced by the Sample Average Approximation, deriving upper estimates of its outcomes dependent on the sample size. The estimates allow to conclude immediately convergence rates for the optimal value of the Sample Average Approximation. As a crucial point the investigations are based on a new type of conditions from the theory of empirical processes which do not rely on pathwise analytical properties of the goal functions. In particular, continuity in the parameter is not imposed in advance as often in the literature on the Sample Average Approximation method. It is also shown that the new condition is satisfied if the paths of the goal functions are Hölder continuous so that the main results carry over in this case. Moreover, the main results are applied to goal functions whose paths are piecewise Hölder continuous as e.g. in two stage mixed-integer programs. The main results are shown for classical risk neutral stochastic programs, but we also demonstrate how to apply them to the sample average approximation of risk averse stochastic programs. In this respect we consider stochastic programs expressed in terms of mean upper semideviations and divergence risk measures. |
||||
16:40 - 17:10 | Coffee break | ||||
17:10 - 17:50 | Kees Oosterlee (Utrecht University) On a robust deep learning methods for fully coupled FBSDEs (abstract)In this presentation, we propose a deep learning based numerical scheme for strongly coupled FBSDEs, stemming from stochastic control. It is a modification of the deep BSDE method in which the initial value to the backward equation is not a free parameter, and with a new loss function being the weighted sum of the cost of the control problem, and a variance term which coincides with the mean squared error in the terminal condition. We show by numerical examples that a direct extension of the classical deep BSDE method to FBSDEs, fails for a simple linear-quadratic control problem, and motivate why the new method works.Under regularity and boundedness assumptions on the exact controls of time continuous and time discrete control problems, we provide an error analysis for our method. We show empirically that the method converges for different problems, one being the one that failed for a direct extension of the deep BSDE Method. 17:50 - 18:00 | John Schoenmakers (WIAS Berlin) | Concluding remarks 18:30 | Dinner |
|
Venue
The workshop takes place in the Erhard-Schmidt lecture room at Weierstrass Institute, Mohrenstrasse 39, 10117 Berlin, Germany. (How to get there)
The lecture room is equipped with a blackboard and a digital projector as well as intranet and internet access.
Accessibility, and day care for children
The venue is wheel-chair accessible by appointment. Kindly inform us about any particular needs. We can provide and sponsor child care during the workshop. Please do not hesitate to contact us.
Contact and further information
Email: Schoenmakers2024@wias-berlin.de
Support
We gratefully acknowledge support by Weierstrass Insitute Berlin (WIAS)