August 23, 2021 - August 27, 2021
Venue: Schloss Dagstuhl - Leibniz-Zentrum für Informatik GmbH

In this school, fundamental and advanced aspects of ML methods were presented with the aim to enable the participants to apply such methods to their specific research problems. In particular, the addressed topics included
- Fundamentals of statistical learning theory
- Introduction to machine learning with python
- Signatures and learning of time-invariant features of time series
- Data driven modeling of dynamical systems
- Distributed learning
- Robust methods for machine learning and data-driven decision-making
- Kernel methods
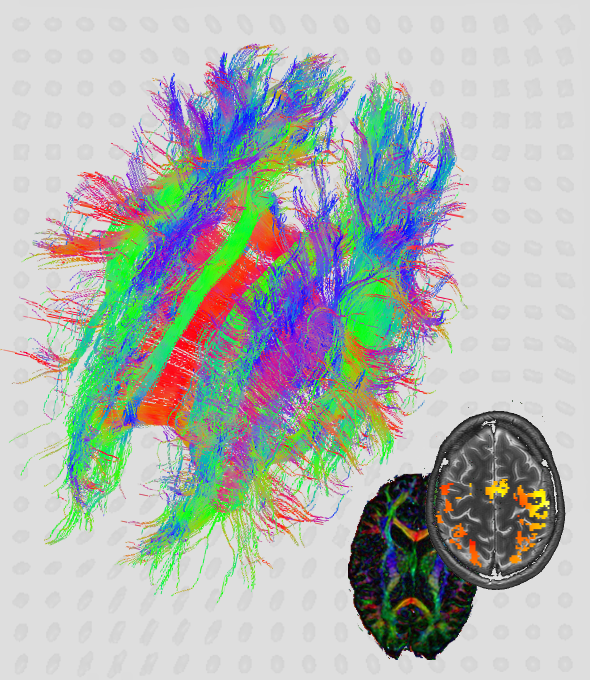

The morning sessions were generally devoted to theoretical introductions to the topics mentioned above, which were then practiced in applied lab sessions prepared and supervised by the speakers in the afternoons. The goal of the summer school was to give the participants a practical entry into the vast field of machine learning underpinned by understanding of the underlying theoretical concepts.
In addition, the summer school provided opportunities for exchange between students and networking, especially important due to the limitations because of the COVID 19 pandemic. In fact, it was the first summer school or workshop with personal attendance for many of the participants.
Particular thanks go to the speakers
- Christian Bayer (WIAS, organizer)
- Martin Eigel (WIAS, organizer)
- Nicole Mücke (TU Braunschweig)
- Feliks Nüske (Paderborn University)
- David Sommer (WIAS Berlin)
- Nikolas Tapia (WIAS Berlin)
- Jia-Jie (JJ) Zhu (WIAS Berlin)
Image copyrights: